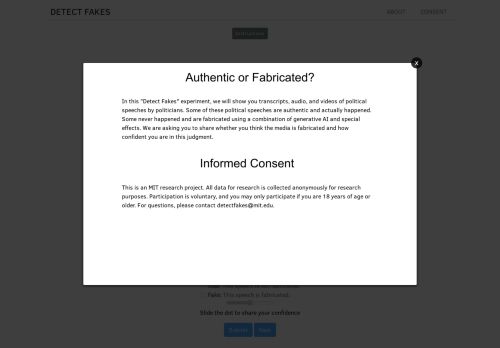
MIT Deep Fakes
Can you spot the DeepFake video?
Can you tell the difference between a deepfake video and a normal video? Find out how good you are at discerning AI manipulated content. See how you rank compared to others after your 10th guess. This page displays two videos: one deepfake and one real (unaltered) video. Your task is to select the deepfake video (the video that has been altered by artificial intelligence). The deepfake manipulation is always a visual manipulation of a face. Sometimes the DeepFake is very hard to detect. Other times, both videos look manipulated. There is always one DeepFake and one real video, and by looking through these videos side by side, you can see how well you do at discerning fake videos from real. After you guess which one is fake, we will highlight which video was which and also tell you which is which. A red border means fake (that’s the correct choice) and a white border means normal (that’s the incorrect choice). This is an MIT Media Lab research project focused on the differences between how human beings and machine learning models spot AI-manipulated media. The question is are humans better at spotting fakes than machines? Or, is it the other way around. Or better yet, are there some kinds of things humans excel at and others that machines excel at? If so, what is that exactly? Our goal is to communicate the technical details of DeepFakes through experience. We hope these DeepFake videos from the recent Kaggle DeepFake Detection Challenge (DFDC) give you a better sense of how algorithms can manipulate videos and what to look for when you suspect a video may be altered. Learn more about DeepFakes and these videos here.Google Talk to Books
Semantic Experiences
Experiments in understanding language
How It Works
How does a computer understand you when you talk to it using everyday language? Our approach was to use billions of lines of dialogue to teach an AI how real human conversations flow. Once the AI has learned from that data, it is then able to predict how likely one statement would follow another as a response. In these demos, the AI is simply considering what you type to be an opening statement and looking across a pool of many possible responses to find the ones that would most likely follow. The technique we’re using to teach computers language is called machine learning. Google’s Machine Learning Glossary defines machine learning as: “…a program or system that builds (trains) a predictive model from input data.” What does that mean for us? Input data: The input data is a billion pairs of statements, where the second statement is a response to the first one. Predicting: We are predicting the response to a question or a statement. After seeing all those pairs of sentences and responses, the AI learns to identify what a good response might look like. Model: The trained system that is used for making predictions. After training, our model is able to pick the most likely response from a pool of options.Talk to Books
In Talk to Books, when you type in a question or a statement, the model looks at every sentence in over 100,000 books to find the responses that would most likely come next in a conversation. The response sentence is shown in bold, along with some of the text that appeared next to the sentence for context.Quick Draw with Google
Can a neural network learn to recognize doodling?Help teach it by adding your drawings to the world’s largest doodling data set, shared publicly to help with machine learning research.
My Goodness at MIT
There are over one million registered charities in the United States alone, and many more worldwide. How do you choose among them? MyGoodness is a simple game that helps you understand how you give. In the game, you will make 10 giving decisions. Each decision is between two choices, and you tell us which you prefer. At the end of the game, we give you a summary of your ‘goodness’ and how it compares to others. You can share that feedback with whomever you would like.